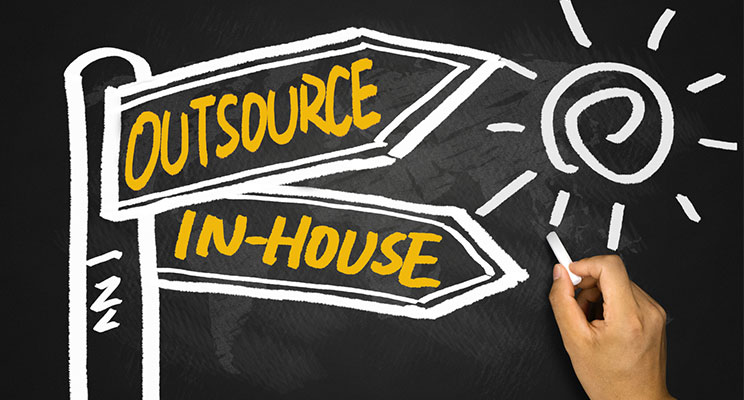
A Tech Reboot: Why Are Fashion Companies Building Their In House Analytics Competencies?
Here’s why fashion businesses have moved from the traditional outsourcing model to building up their inhouse competencies:
Business Context: Unless you are the Kardashians, you understand your family better than an outsider. You know exactly what the business needs at a given time as opposed to a hired hand. When an activity is outsourced to an ‘expert’ outside the organization, they tend to make decisions without taking the business context into account. For example, a particular store might have lower sales because it had a new store manager and all the employees at that store are not aligned with his/ her objectives and practices. But an outsider looks through various forecasting tools and systems and has decided that a particular line of products, which would otherwise perform well, has to be discontinued.
Helps the business be more agile: Brand preference is no longer a thing. The choices are endless. And everything is needed here and now. But consider a business that has their decisions outsourced. Predictions for the season come in on Day 1. Orders are placed, and the production begins. When the products are displayed on Day 30, they realize that Cyan is in vogue and will be sold out by Day 35. A decision needs to be made on the spot by the store manager. But corporate has centralized operations to a team outside the country. Day 40, the store has completely sold out Cyan and is turning back customers. Day 75, Cyan is no longer in vogue, but the store is filled with Cyan pop-ups and unsold merchandise.
Quicker access to Data: The tech stack is complex, and data resides in multiple silos. The company has invested in so many tools and systems and has hired contractors from those providers as experts. To pull out a simple daily dashboard and present it in a format that is relevant to every store is a massive exercise. Even worse when every store manager wants the insights to be presented the way THEY want it. Compare this to an in-house team that has a system that unifies data from all the different systems and empowers business users to build their own dashboards, the way THEY want it, and you’ll see why outsourcing is not in fashion anymore.
Lower TCO over time: Fashion houses have long been hiring external experts, consultants, and hands to execute campaigns. But there is a problem. The problem of volumes. Executing multiple campaigns is turning out to be an expensive affair. As a shortcut, there are services offered by multiple consultancies and outsourcing companies that allow business to do ‘bare minimum’ marketing. Who bears the brunt of it? The customer. While agencies focus on each customer engagement as an activity, they tend to ignore terms like segmentation and personalization. Corporate CHQ might have contracted them to design fancy looking newsletters or trigger based SMS messages that go out in a set frequency and the customer is pounded with unrelated messages that result in churn at the end of the day. More and more fashion businesses are realizing this and are bearing the upfront cost of analytics software that helps them achieve personalization at scale. And they are now seeing the ROI with more customers engaging with the messaging and increasing spend with specific brands.
Bringing in a data-driven culture – In the world of Amazon, the fashion business is no longer just an art. A business that ignores the science part, is no longer relevant. Data-driven marketing has transformed from an innovative approach to a fundamental part of fashion marketing. Strategies are now built on insights pulled from the analysis of big data, collected through consumer interactions and engagements, to form predictions about future behaviors. This involves understanding the data you already have, the data you can get, and how to organize, analyze, and apply that data to better marketing efforts. Crafting experiences and engagements based on data has now moved to the boardroom and with access to real-time data and insights, even to the store.
For a more detailed view of what some of the leading fashion retailers are doing with their in-house analytics teams, visit our website