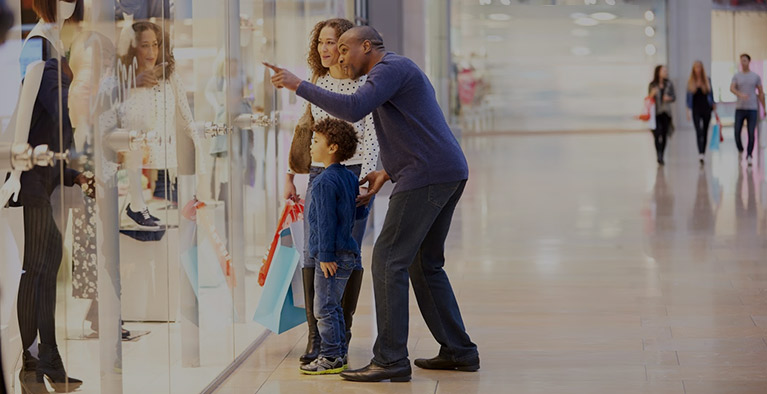
Sort it Out. The need for Customer Centric Merchandising Assortments.
Retailers are under tremendous pressure because of channel proliferation, fickle consumers, increasing price sensitivity and lack of brand loyalty. In order to attract new customers and retain existing ones, retailers must understand customer tastes, trends, buying patterns and tune merchandise assortments accordingly.
Here are a few reasons why building a 'Customer Centric Assortment' strategy is certainly key to Retailer’s success in today’s dynamic environment.
The need for a seamless omnichannel shopping experience
Consumers have become even more demanding than before. Thanks to the internet and social media, consumers are much more aware of what is available, prices across retailers, latest trends; and want the convenience of buying anytime and anywhere across the multiple channels at their disposal. A customer may start browsing products online but choose to buy in store or vice-versa. The number of people shopping online on mobile devices is rapidly growing. A retailer must be reachable across various channels – mobile apps, websites, stores, social media; and be able to recognize and provide a seamless and consistent experience to the customer across channels. In addition, customers have come to expect a personalized experience catered to their tastes, preferences and latest trends as the norm.
Brand Loyalty no more
Customers’ shopping behaviors exhibit “channel-hopping “ which involves looking up products in store, studying reviews and comparing prices before closing in on the “best deal” online. Brand loyalty is under constant attack in developed economies and virtually non-existent in developing economies, especially when buying certain products like fashion and electronics. Customers are both product and technology savvy and extremely fickle. “Social Media influenced buying” is on the rise – heavily influenced by peers groups. In addition, flexibility of payment options is key differentiator for big ticket items as well as aspiration buying.
To be able to succeed it is important to be able to manage operations across multiple channels, have a complete understanding of customer buying behaviours while having a single view of the customer. Thereby, customer centric assortments can directly translate to steeper sales and profitability, stronger brands and more loyal customers.
Learn how you seamless integrate analysis and insights into action and make the shift from Product Centricity to Customer Centricity on this interactive customer centric merchandising page.