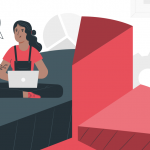
AI powered prescriptive segmentation is hereAutomated customer segmentation for marketing goals
Wouldn’t it be nice if your marketing tool could help define the target segment based on your marketing objectives?
Imagine the current scenario – you and your data scientist spend days, if not weeks, to figure out the characteristics of customers most likely to respond to a given message, and create the list. What if this could be done immediately?
One of the benefits of having a CDP with clean and connected omnichannel data of each customer is that you can start predicting customer behavior with high accuracy. The challenge lies in defining the variables for modeling. This is the value a data scientist brings to the table. She can look at the data profiles, experiment with different variables, and finally, come up with the most relevant variable(s) to feed into the Machine Learning algorithm.
Given the needs of different B2C verticals vary – restaurants, grocery, fashion, specialty, loyalty programs, e-commerce, there can be an infinite combination of measures and filters that must be evaluated.
Sounds tricky? We at Manthan have automated this process.
Our approach dynamically creates multiple combinations of possible variables for each of the verticals, tests for their efficacy and then select the best ones to build the model automatically. Some applications of this model are in look-alike modeling – which can be used for higher cross-sell, upsell and growth marketing. Details on how this is done are here, in a blog by my colleague.
Another ask we often get is around explainable AI. The users aren’t comfortable with black box AI, and we are glad they seek control and want visibility into how the algorithm works. To a marketer, knowing which behaviors are most relevant is key, so other parts of the business can also benefit from the data science.
Let’s take an example, if the algorithm finds that customers who shop across multiple channels are most likely to become stars in the future, they can craft programs that encourage customers to explore new channels. This insight is critical to business growth.
All of this plays out before the campaigns are even executed. Then, there’s the question of returns on campaign spend – how do you know whether the campaigns were effective? Are you able to prove Marketing ROI to your CFO? To aid this, marketers need extensive testing, experimentation, measurement, and attribution capabilities. But, let me reserve that for another piece….